Beyond ChatGPT: An AI-assisted content generation experiment
In the summer of 2023, amid the AI content generation hype, we decided to undertake a project. The goal was simple: to examine the major challenges faced when using AI in content production. More specifically, we were looking to find out whether AI can be used effectively in content creation and to understand the best workflows and tools needed to achieve this.
At Key Content, we focus on delivering first-class, cost-efficient content services to our clients. So, a key part of this experiment involved figuring out how Google responds to AI-generated content with minimal editing on our part. This wasn’t just about solving problems – it was about finding new, exciting, and effective ways to deliver quality content.
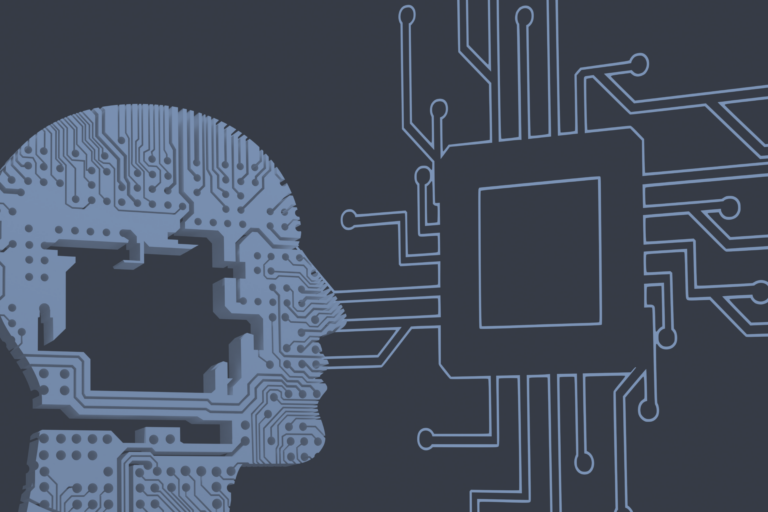
Top challenges of AI-generated content
There were several key challenges we were hoping to address. First up, scalability – we wanted to find out whether it was possible to expand content production efficiently, without sacrificing quality. We also wanted to look at emerging technologies and how AI- assisted content could stay ahead in such a rapidly evolving digital landscape.
Cost-effectiveness was another critical issue, balancing budget constraints to maximise productivity, as well as time management. We needed to ensure streamlining workflows would save time, while still maintaining high-quality output.
Lastly, we looked at the automation of routine processes and SEO optimisation integration, where SEO practices were seamlessly embedded in content across various types and languages.
Goals and objectives
Our goal was to create a content creation process that was not only efficient but also maintained the high standards expected by our clients.
- Exploratory analysis: This is where we started, experimenting with different tools to assess the current capabilities of AI content generation. We also examined different workflows to gauge their impact on the quality of the generated content.
- Error identification: This was another key focus – we wanted to pinpoint the common pitfalls in AI-driven content generation and establish strategies to mitigate them.
- Workflow development: We focused on constructing a robust workflow to address identified errors and inefficiencies, as well as campaign onboarding.
- Production ramp-up redefinition: We used AI to streamline and enhance the content production ramp-up process.
- Quality framework overhaul: We revamped existing frameworks to include diverse metrics and considerations taken from our analyses. This is crucial, as AI-assisted content generation needs a new quality framework to capture any potential errors that stem from AI biases.
SEO and AI content: We investigated how Google indexes and ranks AI-generated content with minimal human intervention.
Methodology
1. Defining the process and timelines
Establishing clear case study objectives was key. For the ideation phase, we focused on content type, topics, and categories, and made sure these aligned with our already successfully tested SEO strategy. We then added our usual steps to be able to compare pure human-generated content against the AI-assisted version.
Our next move was to conduct extensive keyword research and keyword mapping. One of the main goals here was to test all the different tools in the fairest way possible. After running keyword research and keyword mapping across different categories and subcategories, we then assigned the same traffic opportunity to all of them and monitored the results. Our methodology also included preparing the website infrastructure for immediate content deployment, as well as setting timelines and creating content publishing schedules.
2. Selecting the top AI content generation tools
For this project, it was particularly important for us to make sure we were choosing the right AI content creation tools, as well as assigning the right content types based on our initial research. We tested a wide range of different tools, including Copysmith, RightBlogger, Kafkai, Writesonic, ChatGPT, and Jasper.
3. Design workflows
Several key considerations needed to be taken into account when designing workflows. First, we put together a resources plan, before moving on to producing a range of content with a variety of different AI tools. Then we needed to carry out minimum editing work to see how the output performed in the SERP. After that, it was time to kick off the project based on our SEO strategy and use SMEs to analyse the quality of the output.
SEO strategy is another essential factor that needed addressing. We had to take a close look at traffic opportunity analysis, category and subcategory definitions, as well as keyword research and mapping.
Our onboarding process started with the creation of a new triage checklist, to define which workflow was going to be assigned to each different content type (e.g. blog articles, social media ads, Google Ads, e-commerce products). We then created the onboarding plan, including the AI-assisted tasks to work on an initial small batch of content. Once we’d defined the feedback loop and adjusted the workflow, it was time to implement a ramp-up plan with the delivery schedule.
When it came to content production, we decided to develop tailored workflows for each selected tool and document our key learnings. We then implemented staggered content production and published over 200 articles in two months. We waited for the content to start being indexed and then published another round of 200 articles. Following that, we discarded any content that failed to meet our quality standards. Finally, we reviewed and edited the processes, incorporating SEO checklists and common error rectification.
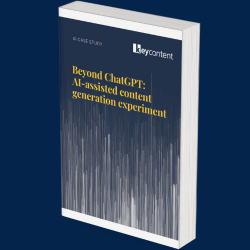
Download the whitepaper
Access the full results and findings in the downloading the full case study of our AI content experiment.
Summary of results
Organic performance
When it came to organic performance, we encountered some issues:
- The organic visibility analysis showed that Google identified the content as neither helpful, nor following the EEAT guidelines.
- Our SEO strategy was correct, but as one of our requirements for this test was to do a minimum edit on the content, the results didn’t meet the standards of most of the tools.
- The instructions needed to be followed in scale, and the optimisation work we carried out in the initial phases was lost when we scaled the amount of content produced by AI tools.
Key Learning: We identified the key factors that help AI-generated content rank better, such as content length, structure, keyword optimisation, and uniqueness. We concluded that human review steps and AI fine-tuning are still needed to ensure the content meets the right standards.
Content production enhancements
We encountered many benefits of integrating AI into the content creation process, including consistency, cost-effectiveness, and adaptability. We also uncovered many AI training benefits for future content creation campaigns.
We are also still looking at other integrations to be able to publish quicker from the final outputs, while general issues with compatibility and integrations remain on most of the tools we checked.
Scalability & quality
Quality Through using a dynamic quality framework, we’ve been able to adjust and fine-tune our results very efficiently. Google performance analysis has shown us that the content has to be unique, following an intention and giving the user all the information that is required. If the content is too short or not personalised, or if it lacks authority, it is detected and ignored.

Key insights
-
-
- If we change how we do things, the workflows have to change. This includes the way we measure quality. The crucial role of AI in supporting content creation emphasises the need for well-defined workflows and strategic human intervention.
- The only way to be efficient is to be able to integrate feedback into the instructions for AI-generated content. This shows the importance of a feedback loop for continuous improvement and to avoid repetitive errors.
- Even if you use humans for the strategic steps, if the AI system is not well implemented, the strategy won’t work. Strategic human steps are always needed, but if the AI content creation process is not properly set up, the output will be low-quality, independent of the SEO analysis done.
-
Download the case study
Fill the form to get full results on AI-assisted content creation experiment
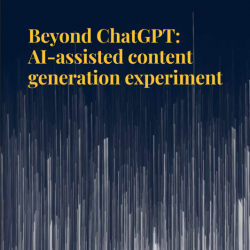